Despite what late 20th-century sci-fi pop culture taught us, robots are yet to take over the world. But it hasn’t all been false promises: machine learning now permeates almost every echelon of society. Computers and AI systems are becoming proficient in a diverse range of tasks across multiple industries. The seven machine-learning applications we have outlined in this guide are just the proverbial tip of the iceberg. Let’s take a look.
Believe that AI and digital are our future? Join BeInCrypto Trading Community on Telegram: of crypto-lovers on Telegram: learn more about the currency of the future and decentralized systems, start trading with our FREE Trading Basics course and discuss coin trends with PRO traders! Join now
What is machine learning?
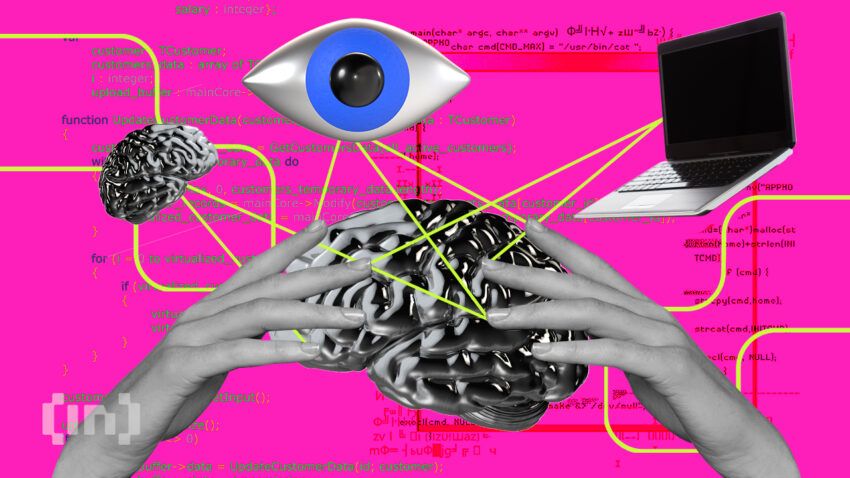
Put simply, machine learning is a powerful and pervasive form of artificial intelligence (AI). It is an application of AI that allows computers to learn from experience and improve themselves without requiring further programming.
Arthur Samuel, a pioneer in the field of computer gaming and AI, first coined the term “machine learning” in 1959. He defined machine learning as a “field of study that gives computers the ability to learn without being explicitly programmed.”
Machine learning uses data and algorithms to mimic human learning. The goal is to become increasingly accurate while making classifications, predictions, or fetching data-driven insights. Broadly speaking, it operates in three basic ways:
- Using a combination of algorithms and data to predict patterns and classify data sets
- Evaluate accuracy using an error function
- An optimization process to fine-tune the data points to generate the desired model
3 types of machine learning
Machine learning has three further sub-categories:
- Supervised learning
- Unsupervised learning
- Reinforcement learning
The following is a rundown of each of these sub-categories.
Supervised learning
As the moniker suggests, supervised machine learning requires some degree of human oversight. It requires a known set of input and output data during the training phase of the machine learning lifecycle.
Generally, a data scientist labels the training data before using it to train and test the model. Once the model learns to establish a relationship between the input and output data, it goes on to classify unknown datasets to predict outcomes.
Most data in this scenario is raw and unlabeled. Human “supervision” is required for the data to be accurately labeled, so the model can identify a correlation that connects the input and output data. This is often a very resource-intensive process involving gigantic volumes of raw data.
Supervised learning comes in handy for use cases such as:
- Inventory optimization
- Identifying risk factors for diseases
- Evaluating loan applications to find out whether an applicant is a low risk or high risk.
- Detecting fraudulent transactions
- Predicting real estate prices
Unsupervised learning
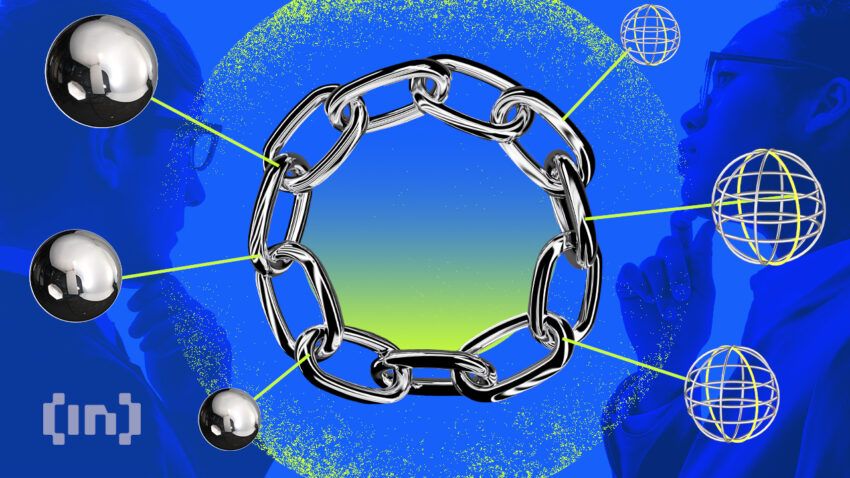
In unsupervised machine learning, models are trained on raw and unlabeled data. This method is useful when identifying patterns in raw datasets or clustering similar data into groups.
Unsupervised learning is also appropriate if an early exploratory phase is necessary to better understand the datasets. Unlike supervised learning, this approach requires little to no human oversight. Usually, a data scientist defines the model hyperparameters. However, the model then proceeds to independently process huge arrays of data effectively.
Because of the nature of its approach, unsupervised machine learning has been very successful while finding patterns and trends in raw datasets. It is best for applications such as:
- Classifying customer profiles as per their purchase or consumption behavior.
- Classifying inventory items according to manufacturing and/or sales metrics.
- Highlighting associations in customer data. For example, customers who bought specific types of clothing might be interested in specific types of shoes.
Reinforcement learning
Reinforcement learning is used to train machine learning models to make a series of decisions sequentially. The objective is to teach the model to achieve the desired outcome in an uncertain and potentially complex environment.
In this approach, the model is subject to a game-like environment. It is required to deploy a trial-and-error method to come up with a solution.
To achieve the desired outcome, the model gets either rewards or penalties based on the actions it performs. For the machine learning model, the goal is to maximize the total reward.
Even though the designer determines the reward/penalty policy, they do not actively interfere in how the model goes on to solve the problem. It is up to the model to figure out how to deliver the desired outcome and maximize its rewards. That is where it differs from supervised learning.
Generally, this approach begins with the model starting with a random trial-and-error method and eventually finishing with sophisticated tactics.
A couple of examples of reinforcement learning’s real-life applications include:
- Training autonomous vehicles to drive and park themselves without requiring human intervention.
- Operating traffic lights dynamically to help control traffic.
Top 7 machine learning applications
1. Diagnosing diseases
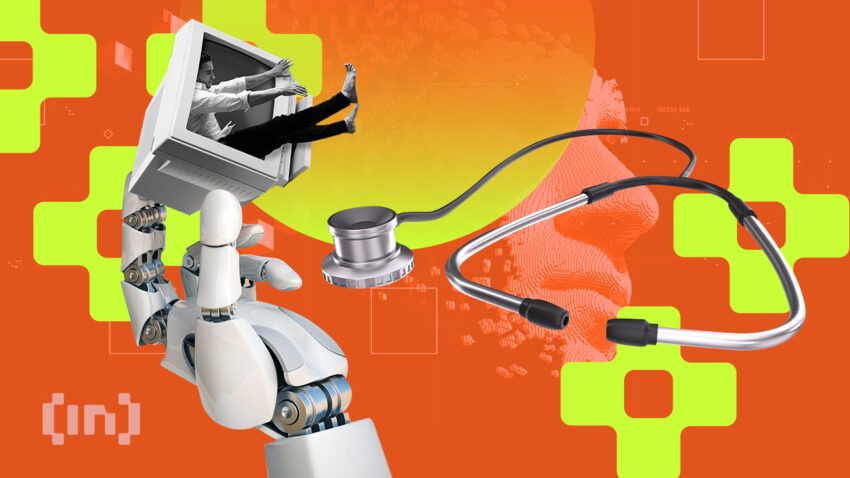
One of healthcare’s main machine learning applications is identifying and diagnosing otherwise hard-to-diagnose diseases. Typical examples include various types of cancer that are tough to detect during the initial stages and genetic diseases.
For example, the now-defunct IBM Watson Genomics gave a very good glimpse at how combining genome-based tumor sequencing with cognitive computing can lead to a significantly faster diagnosis. Similarly, a leading biopharma company, Berg, has already deployed machine learning to develop therapeutic treatments in various domains, including oncology.
Another good example of machine learning applications in the healthcare sector would be P1Vital’s PreDicT program, which stands for Predicting Response to Depression Treatment.
2. Image recognition
Image recognition is all around us. Notice the automatic scene and facial recognition feature in your smartphone’s gallery app? That’s done with machine learning. The same goes for social media features such as automatic friend tagging. Take Facebook’s Deep Face technology, which uses machine learning to offer suggestions to tag friends in pictures. State-of-the-art secret surveillance programs deployed by some world governments also rely on image recognition facilitated by machine learning.
Image recognition with machine learning also has applications in the healthcare sector. During many treatment procedures, missing even a tiny element could be devastating. Scans and X-ray pictures can use image detection to detect the tiniest of changes from one point to the other. This technique is particularly useful to accurately detect the progression of a virus infection or the spread of a tumor.
3. Autonomous vehicles
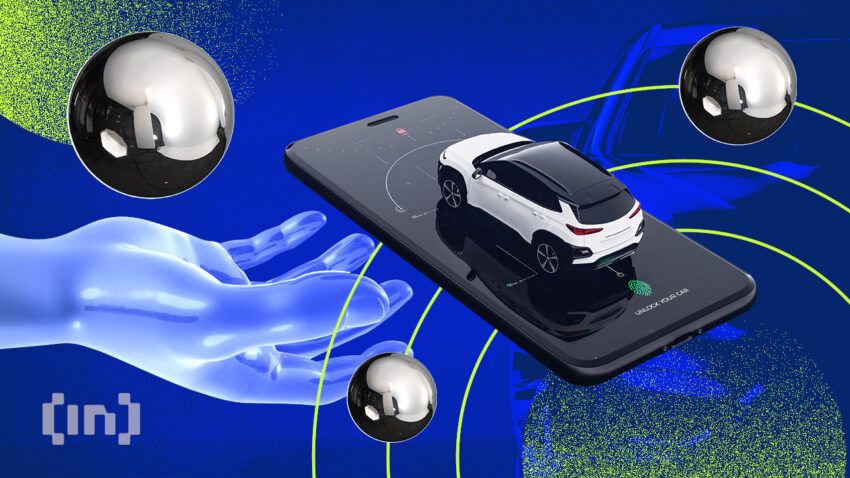
With autonomous vehicles, AI is expected to outperform a human driver, regardless of the scenario. And that’s precisely where machine learning algorithms come into play. Along with deep learning, machine learning algorithms allow autonomous vehicles to make decisions in real-time.
The application of machine learning in self-driving cars like the Tesla Model S or Ford F-150 involves the onboard computer taking data input from a raft of sensors. This is to ensure that the ADAS can safely and accurately make sense of the world around the vehicle. The system uses this data stream to control the vehicle’s speed and direction. Additionally, the data is used to process other key activities like object detection and tracking, perception, and prediction.
4. Online fraud detection
Machine learning is increasingly useful in securing our online transactions and detecting forgery. It is especially effective at detecting fraudulent behavior involving the use of fake accounts, fake IDs, and phishing.
The application of machine learning in fraud detection involves algorithms trained with historical data to suggest risk rules. These rules can be implemented to block certain user actions that are flagged as suspicious — for example, suspicious logins, possible identity thefts, fraudulent transactions, and so on.
Usually, data scientists feed the machine learning model a huge volume of records of previous fraud and forgeries. They also flag non-fraud cases to eliminate false positives as much as possible. The higher the volume of data, the more accurate the rule suggestions generally are.
5. Product recommendation
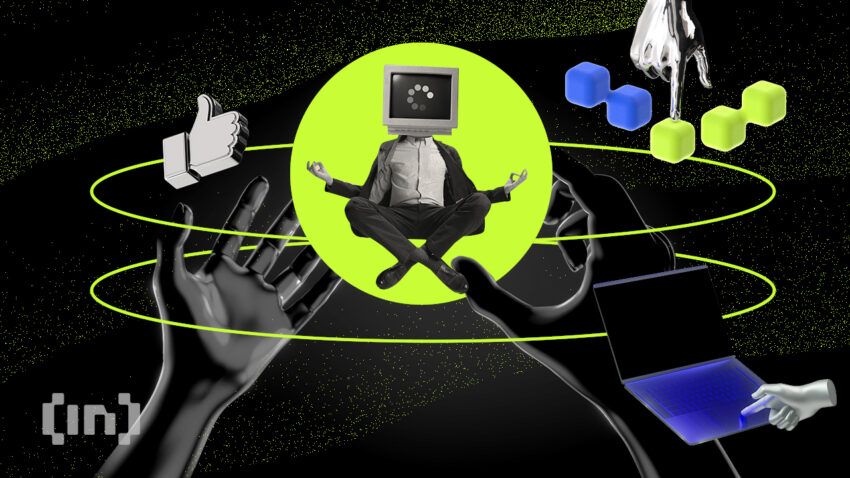
Product recommendations on e-commerce websites and entertainment platforms are prime examples of machine learning applications in business.
These recommendations are generated and delivered by “recommender systems.” These powerful engines leverage machine learning algorithms to segment customers as per their user data and behavioral patterns. User data and behavioral patterns are harvested from browsing history, likes, shares, and so on.
Market segmentation variables typically include:
- Behavioral: purchase patterns, usage rate, history, and loyalty status
- Demographic: age, gender, income
- Geographic: city, region, climate, etc
- Psychographic: interest, personality, lifestyle
Each consumer is served with personalized recommendations, content suggestions, and ads based on these variables.
6. Intelligent Virtual Assistant (IVA)
IVAs are machine learning-based systems capable of understanding natural language (read: human language) and responding accordingly. These intelligent AI assistants present a multiple-choice selection of answers to each question. They can also understand user intent from free text.
IVAs are increasingly finding their use in customer support automation, i.e., Virtual Customer Assistants. This allows users to articulate even highly specific concerns to the machine as they would explain to a human.
Note that IVAs are not the same thing as chatbots. They are much more sophisticated and capable of understanding natural language. In comparison, most chatbots communicate with users by offering simple yes/no types of options.
With the rise of no-code products, it is now possible to build IVAs in as little as 15 minutes.
7. Trading
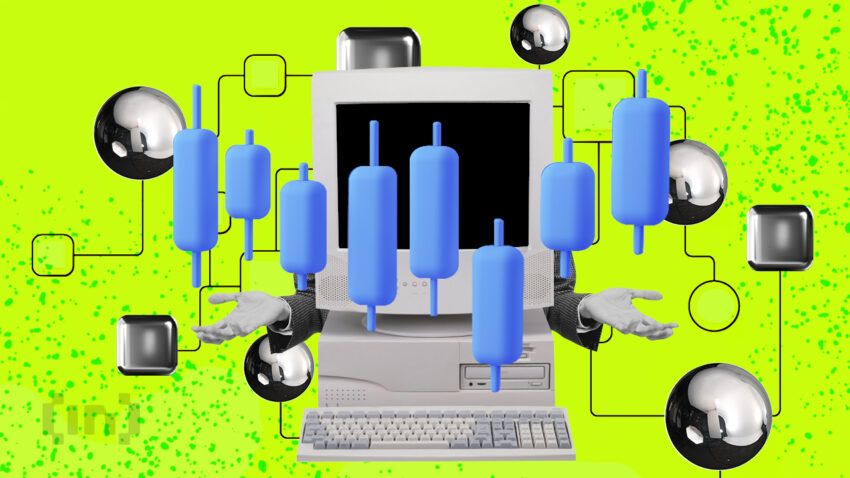
Machine learning also has growing applications in stock and crypto trading. Sophisticated machine learning algorithms do the under-the-hood calculations and analyses. The data generated helps traders make informed decisions.
More specifically, machine learning comes in handy when it comes to extracting signals from financial and alternative data. These signals can then be used to design and backtest systematic strategies.
What does the future hold?
Machine learning applications are finding a place in most aspects of our society. This guide simply listed a few examples.
Other common applications include sentiment analysis; collecting and classifying data in cosmology; marine life studies; engineering; military intelligence; and even digital art. Artificial intelligence is unlocking more technological advancements with its growing reach every day. It’s only sensible to educate ourselves on this technology as it grows in importance and relevance.
Frequently asked questions
What are the applications of machine learning?
What are machine learning types?
What is a real-life example of a machine learning application?
Disclaimer
In line with the Trust Project guidelines, the educational content on this website is offered in good faith and for general information purposes only. BeInCrypto prioritizes providing high-quality information, taking the time to research and create informative content for readers. While partners may reward the company with commissions for placements in articles, these commissions do not influence the unbiased, honest, and helpful content creation process. Any action taken by the reader based on this information is strictly at their own risk. Please note that our Terms and Conditions, Privacy Policy, and Disclaimers have been updated.
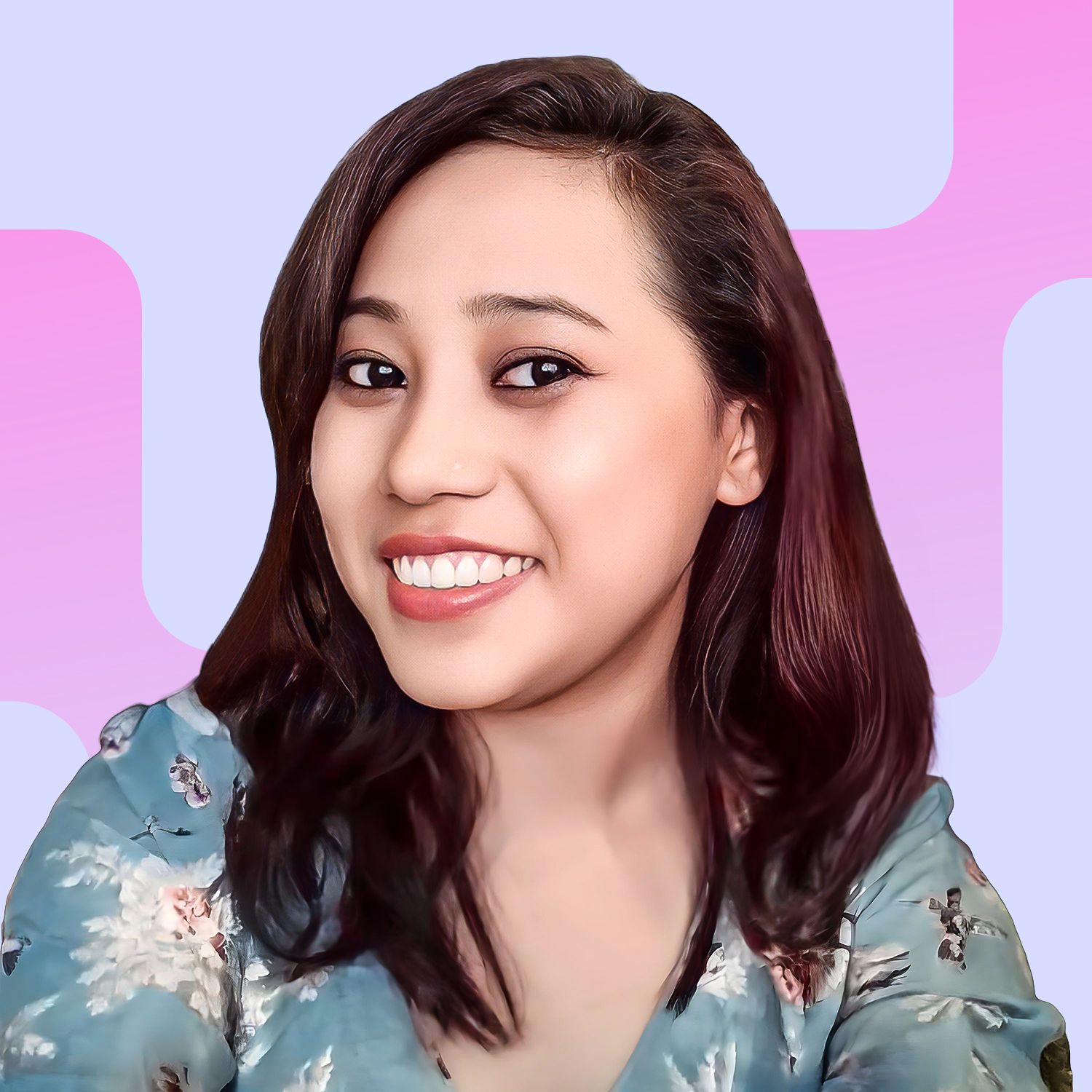